The management of Big Data has become a decisive challenge for companies, which need to have a high level of visibility over the flow of data produced, and to be able to respond to specific needs linked to different business lines. In a wide variety of business contexts, access to specialized data ordered according to criteria defined by users and business specialists is becoming essential, and can provide decisive competitive advantages.
The Datamart is the tool that meets this need: that’s why companies are multiplying their strategic Datamarts.
What is a Datamart?
The Datamart, or data store, has become an essential tool within a growing number of companies to ensure the rapid processing of data by their business experts.
The strength of the Datamart lies in the fact that it gathers specialized data intended for specific professional activities. Professionals can access it and quickly find, in an organized form, the information they need to make decisions, develop business strategies, and more. The Datamart can be considered a subset of a Data Warehouse intended for specific categories of users.
Indeed, while the Data Warehouse collects all the raw data produced by a company to sort and organize it, the Datamart contains sorted, aggregated, and organized data according to business uses or specific domains. It is accessed by professionals with well-defined and pre-known needs.
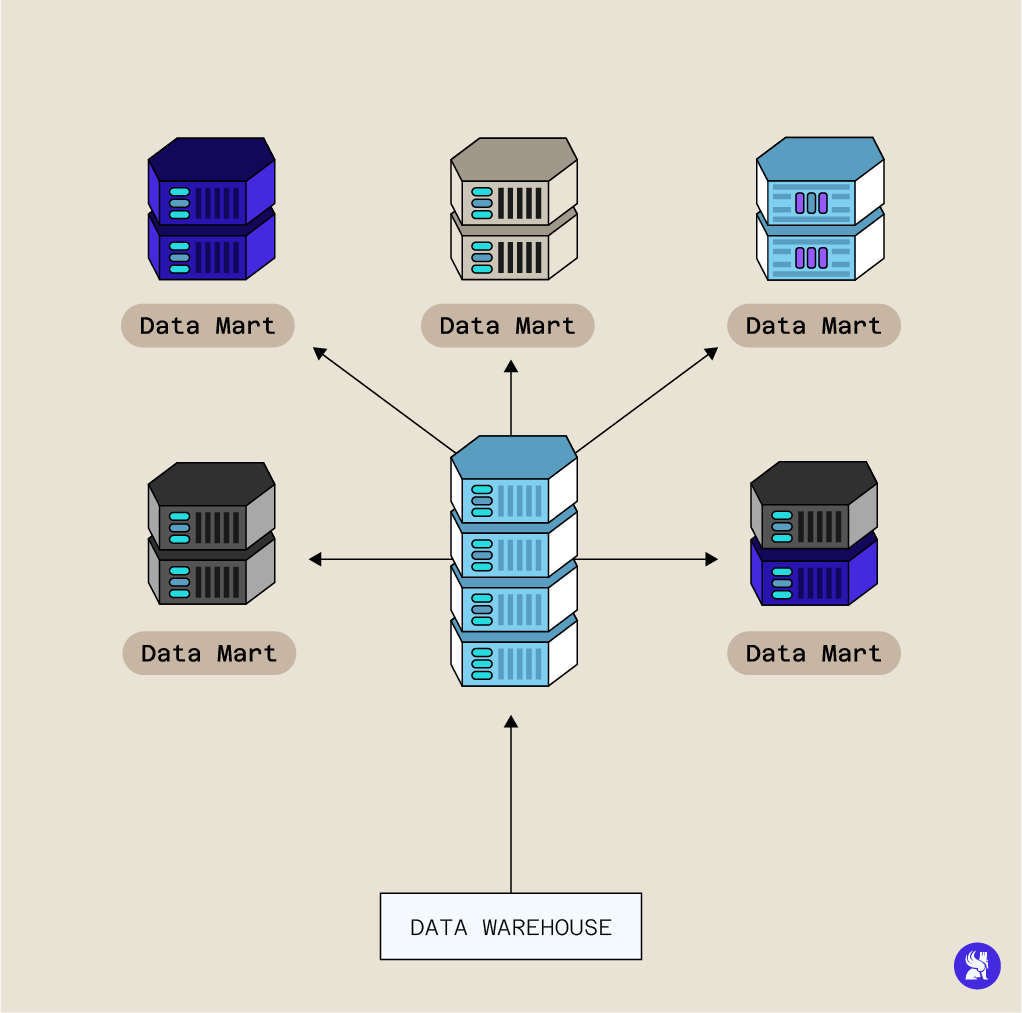
What are the different types of Datamart?
The operation of the datamart has been theorized according to two different schools of thought by two computer science researchers: Bill Inmon and Ralph Kimball. The difference between the two schools is the positioning of the datamart within a company’s databases.
According to Bill Inmon, the datamart corresponds to a data stream that comes from the Data Warehouse and is sorted according to specific requirements. Therefore, the Datamart contains specialized data intended to be used by business experts.
For Bill Inmon, the Datamart occupies a peripheral position to the Data Warehouse. In contrast, according to the approach proposed by Ralph Kimball, the datamart is at the very core of the Data Warehouse. In other words, according to this approach, the Data Warehouse itself is composed of several datamarts that group aggregated specialized data.
Both approaches converge in a vision of the datamart as a specialized and organized subset of a Data Warehouse.
Datamarts can be classified into three groups based on their relationship with the Data Warehouse. We find dependent datamarts, independent datamarts, and hybrid datamarts.
- The dependent datamart is strictly connected; it was created from the Data Warehouse, representing a subset of it.
- The independent datamart was not created from the Data Warehouse, and its source may be different.
- Finally, the hybrid datamart allows the integration of sources from both the main Datamart and other operational systems.
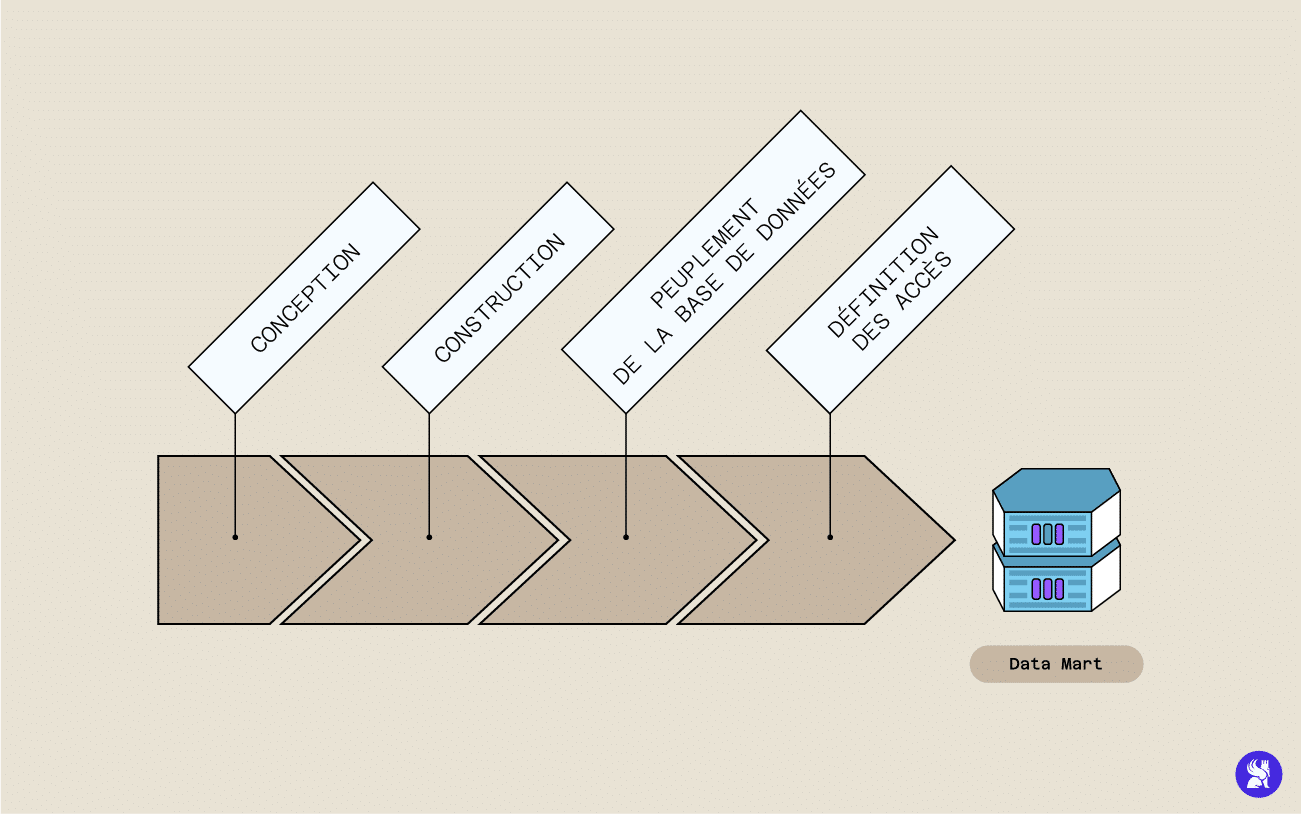
Datamart structure and benefits
Datamarts can be structured using different schemas, with the most popular being the star schema and the snowflake schema. The star schema has the advantage of requiring fewer joins when writing queries because there is no dependency between dimensions. On the other hand, the snowflake structure requires less storage space but has a more complex architecture.
Datamarts offer significant advantages that have contributed to their popularity. First, they allow working with smaller and more coherent portions of data. This makes searching and analyzing data easier and faster.
Furthermore, by organizing data into multiple specialized blocks and isolating them from their sources, congestion in the Data Warehouse can be avoided. With such a setup, different professionals within the same organization can find the information they need in the Datamart dedicated to their field of work instead of searching in the Data Warehouse.
Moreover, thanks to this organization, users can have quick access to diverse data, knowing in which Datamart it is categorized. Due to its organization and reduced dimensions, managing and maintaining a Datamart is much faster and simplified compared to managing a Data Warehouse.
Another advantage of the Datamart is its user-friendliness. End-users can easily access information without necessarily needing to have knowledge of the entire Data Warehouse or compile complex queries.
Finally, the fact that data is aggregated and organized based on predefined criteria allows for quick analysis of key trends and, therefore, the ability to quickly adopt operational strategies.
How do you build a Datamart?
Often, a Data Scientist can work with a pre-established datamart. Other times, they may need to create one to facilitate data processing and decision-making within their company.
Being able to harness the necessary skills to create a Datamart is an important asset for an experienced Data Scientist or a beginner. To create a Datamart, a Data Scientist can proceed in stages.
Firstly, they must design a robust, accessible, and functional Datamart. To do this, they need to identify both the data produced by the company, their various sources, and the key needs of different departments. They then define the subsets in which to group the data, namely their basic schema. They subsequently organize the logical layout of the schemas and their physical structure.
After the design work, the Data Scientist begins to build the database and its logical structure. It is during this stage that they create tables, indexes, and access controls.
The third step is to populate the datamart by transferring data from various sources. The Data Scientist must be careful to clean and organize the data before integrating it into the datamart.
The fourth step involves creating a structure that allows easy and functional access to the data for business experts. Optionally, the Data Scientist can configure an API or interfaces to facilitate data usage and access.
Finally, the Data Scientist must manage the Datamart by controlling access, adding relevant new data, and handling failures.
By training for the role of a Data Scientist, you will learn how to leverage these skills to enhance data management within your company.