This article is the first in a series dedicated to Deep Learning: After a general introduction to the functioning and applications of neural networks, you will discover in the following articles the main types of networks and their architectures, as well as methods and various examples of applications of Deep Learning today.
Let’s start our Introduction to Deep Learning.
Key concepts: AI, Machine Learning, and Deep Learning
In recent years, a new lexicon related to the emergence of artificial intelligence in our society has flooded scientific articles, and it is sometimes difficult to understand what it is. When we talk about artificial intelligence, we often refer to associated technologies such as machine learning or deep learning. Two terms that are extremely used with more and more applications, but not always well-defined. To begin, let’s go back to these three essential definitions.
- Artificial intelligence: is a field of research that groups together all the techniques and methods that tend to understand and reproduce the functioning of a human brain.
- Machine Learning: is a set of techniques that give machines the ability to automatically learn a set of rules from data. Unlike programming, which consists of executing predetermined rules.
- Deep Learning : is a machine learning technique based on the neural network model: dozens or even hundreds of layers of neurons are stacked to bring more complexity to the establishment of rules.
Machine Learning: Supervised and unsupervised learning
Machine Learning is a set of techniques that give machines the ability to learn, as opposed to programming, which consists of the execution of predetermined rules.
There are two main types of learning in Machine Learning. Supervised and unsupervised learning.
In supervised learning, the algorithm is guided with prior knowledge of what the output values of the model should be. As a result, the model adjusts its parameters in order to reduce the difference between the results obtained and the expected results. The margin of error is thus reduced as the model is trained, in order to be able to apply it to new cases.
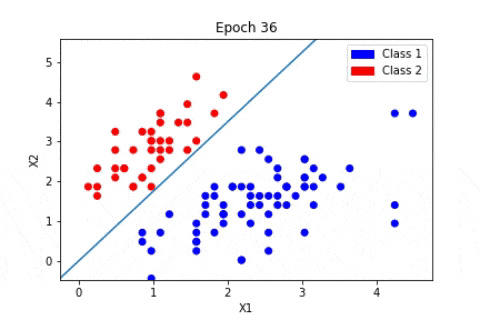
On the other hand, unsupervised learning does not use labeled data. It is then impossible for the algorithm to compute a success score with certainty. Its objective is therefore to deduce the clusters present in our data. Let’s take the example of a data set of flowers, we want to group them into classes. Here, we do not know the species of the plant, but we want to try to group them, for example, if the shapes of the flowers are similar then they are related to the same corresponding plant.
There are two main areas of models in unsupervised learning to find groupings:
- Partitioning methods: k-means algorithms.
- Hierarchical clustering methods: hierarchical ascending classification (HAC)
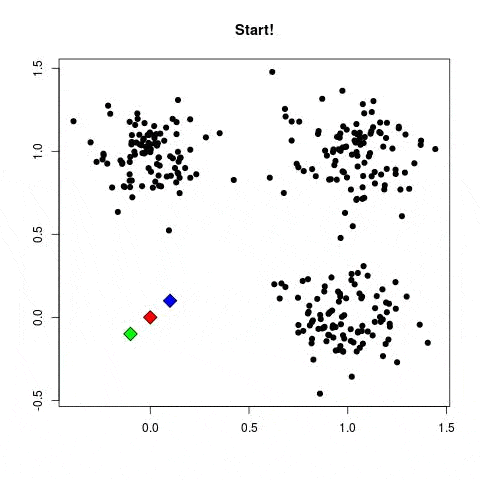
What is Deep Learning?
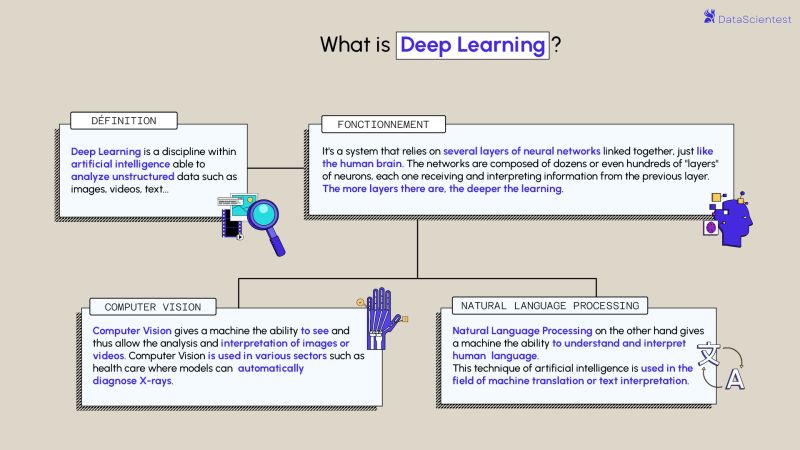
Deep learning is one of the main technologies of machine learning. With Deep Learning, we are talking about algorithms capable of mimicking the actions of the human brain thanks to artificial neural networks. The networks are composed of dozens or even hundreds of “layers” of neurons, each receiving and interpreting the information of the previous layer.
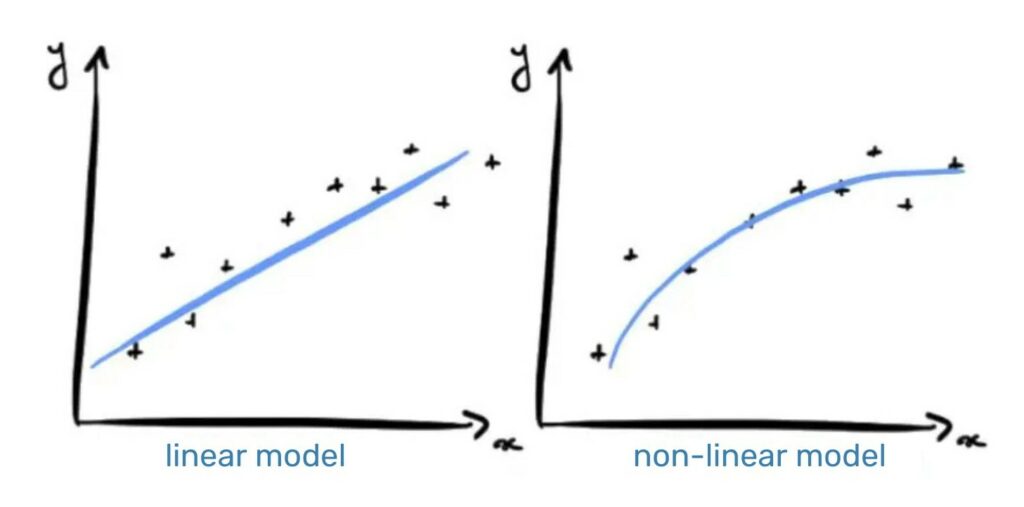
Each artificial neuron represented in the previous image by a circle, can be seen as a linear model. By interconnecting the neurons in a layer, we transform our neural network into a very complex non-linear model.
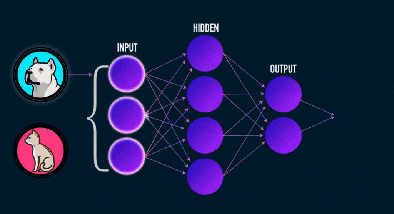
To illustrate the concept, let’s take a problem of classification between a dog and a cat from images. During the learning process, the algorithm will adjust the weights of the neurons in order to reduce the gap between the results obtained and the expected results. The model will be able to learn to detect triangles in an image, since cats have much more triangular ears than dogs.
What is Deep Learning used for?
Deep learning models tend to work well with large amounts of data, whereas more traditional machine learning models stop improving after a saturation point.
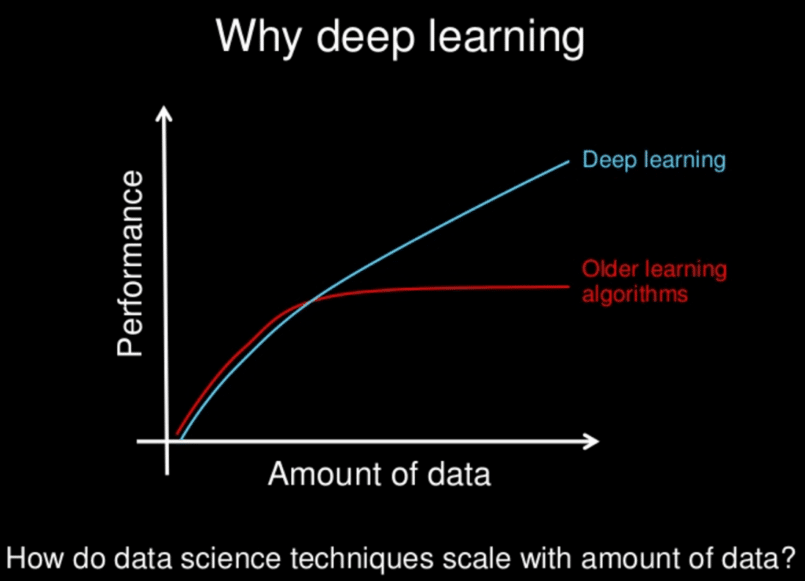
Over the years, with the emergence of Big Data and increasingly powerful computer components, power- and data-hungry Deep Learning algorithms have outpaced most other methods. They seem to be ready to solve many problems: recognizing faces, defeating poker or go players, enabling the driving of autonomous cars or even searching for cancer cells.
AI in the professional World
Almost every industry is affected by AI. Machine Learning and Deep Learning play a big role in this.
Whether you are a medical professional or a lawyer, it is possible that one day a highly autonomous model will assist you or even replace you. In the healthcare industry, there are already applications to automatically diagnose a patient.
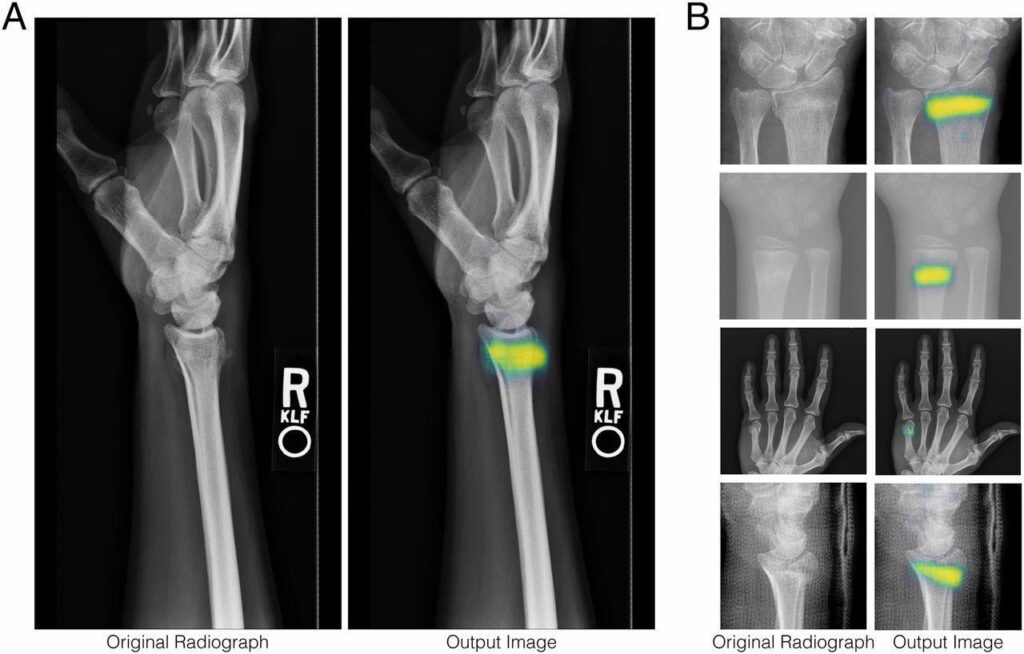
The automotive industry is also being shaken up with the arrival of assisted driving.
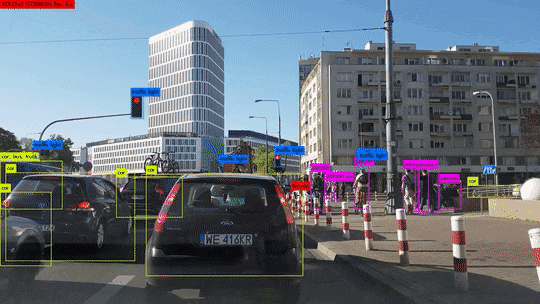
It is also thanks to deep Learning that Google’s Alpha Go model managed to beat the best Go champions in 2016. The search engine of the American giant is itself increasingly based on deep learning rather than on written rules.
Today, deep learning is even capable of “creating” paintings by itself. This is called Style Transfer.
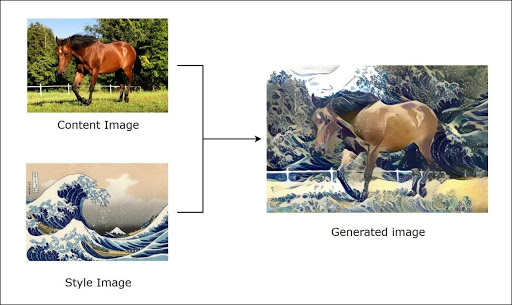
In the following part of the article, we will introduce you to neural networks with a new approach, we hope you will appreciate it!
Deep Learning as a solution in e-commerce
AI analysis used in e-commerce
It is obvious that the e-commerce industry generates large amounts of data. Companies, merchants and retailers are aware that Big Data solutions to manage their operations will make their business more valuable. Despite the irruption of all these innovative solutions, Big Data can be a blessing or a curse, depending on how it is used and applied.
The artificial intelligence revolution aims to make it easier to manage this huge amount of data, thanks to intelligent technologies such as deep learning. It is essential because it provides elements for better data analysis.
In a practical case, AI analysis makes it easier for an online store to offer interesting products to its customers, to highlight their preferences and to provide them with personalized attention. Deep learning automates what is known as predictive analysis. With this, customers can receive suggestions when making a purchase.
Deep learning defines a style when it comes to e-commerce. It is not about creating online sites that attract large proportions of buyers. The goal is to send clear, individualized messages to each of them.
Big Data is subjected to deep analysis through deep learning, which leads to facilitating the buying process of customers. Deep learning algorithms help the company to get a better experience and keep track of those who have visited its site.
Deep learning is coming in to facilitate the expansion of e-commerce. Online sales are being driven by technology trends such as chatbots.
In a way, Deep Learning is redefining online commerce, and we’re still in its infancy. Therefore, those who adopt it will have more advantages.
Deep Learning and neural networks: biological or artificial?
Before tackling the precise functioning of neural networks, we thought it would be interesting to draw a parallel with biological neurons.
Biological neuron : Structure and role
The nervous system is composed of billions of cells: it is a network of biological neurons. In fact, neurons are not independent of each other, they establish links between them and form more or less complex networks.
The biological neuron is composed of three main parts:
- The cell body is composed of the control center processing the information received by the dendrites.
- The dendrites are the main wires through which the information coming from the outside passes.
The axon is the conducting wire that leads the output signal from the cell body to other neurons.
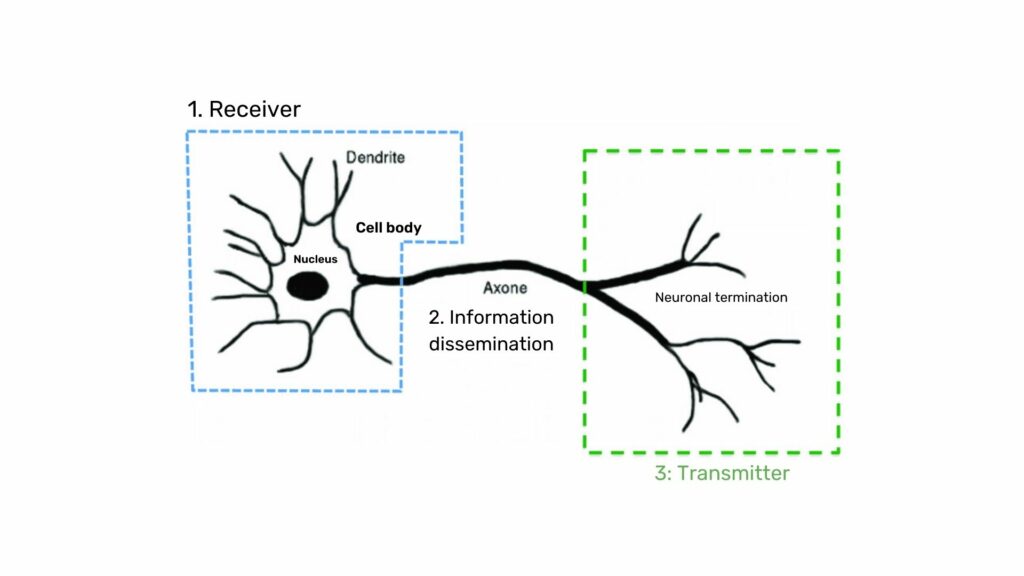
As for synapses, they act as links and weights between neurons and therefore allow neurons to communicate with each other.

What is the link between biological and artificial neurons?
In summary:
Biological neurons have a control center (called a somatic cell) that sums up the information collected by the dendrites. Then, the control center returns an action potential according to the following rules:
- If the input sum does not exceed the excitation threshold: no nerve message via the axon.
- If the input sum exceeds the excitation threshold: a nerve message is sent via the axon (this is the idea, but in reality a little more complicated).
Let’s proceed to a simple comparison of the main steps of the perceptron algorithm with the constitutive elements of biological neurons. This choice of algorithm is justified because it is as close as possible to the functioning of biological neurons:
- Synapses/dendrites: weighting of each element in input wi. xi
- Cell bodies: application of an activation function f to the sum of the weighted inputs
- Axon: output of our model

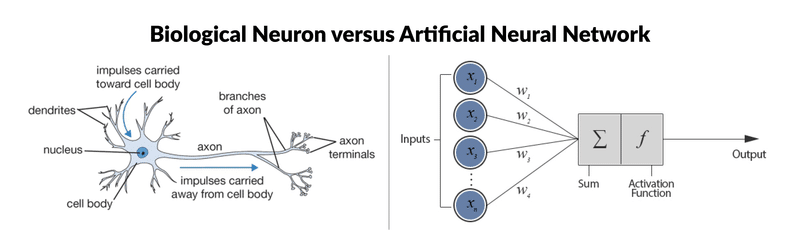
The specific vocabulary for this algorithm is as follows:
- The vector w is called the weight vector (which adjusts during training).
- The vector x is called the input vector.
f is referred to as the activation function.
For most activation functions, the perceptron consists of finding the separating hyperplane (defined by w) between our two classes:

The simple Perceptron algorithm is no longer used in practice, since other algorithms such as the Support Vector Machine are much more efficient. Also, biological neurons are not used individually, they are usually linked to other neurons.
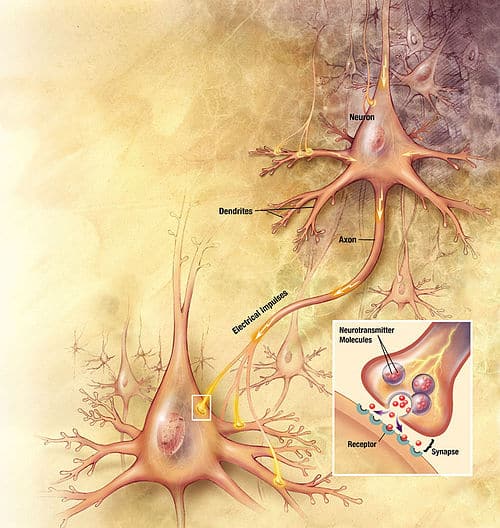
The interest in the Perceptron algorithm comes from a technique demonstrated in 1989 by George Cybenko which consists in linking and stacking layers of perceptron to bring more complexity. An algorithm of this type is called Multilayer Perceptron (MLP).

In the previous figure, the model consists of classifying (in 10 classes) images of handwritten figures. The green squares are the inputs of our model, the perceptrons are represented by gray circles and the links are represented by the arrows.
In general, the last layer of our model is used to format the desired result. Here, as we have a classification problem, we want to predict the probability of each class (number 0, number 1 …). This is why the last layer has 10 neurons since there are 10 classes, and a “softmax” activation function allowing it to return a probability.
It is much freer for the other layers of our model, it is especially important that the activation functions of the perceptrons are non-linear to make the model more complex. In practice, the tanh or ReLU activation functions are the most used.
Like for LEGO’s, it is up to the Data Scientist to choose the architecture of his model.
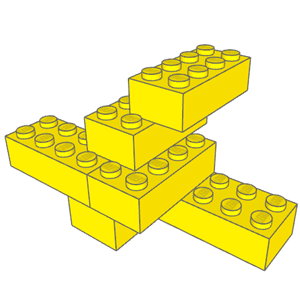
There are architectures that are more efficient than others, but there is no real mathematical rule behind them. It is the experience that prevails on the choice of the model structures.
Sources :
- F. Rosenblatt (1958), The perceptron: a probabilistic model for information storage and organization in the brain
- G. Cybenkot (1989), Approximation by Superpositions of a Sigmoidal Function