Continual Learning opens up new perspectives in the age of constantly evolving artificial intelligence. This approach enables models to adapt gradually to new data without losing the knowledge they have already acquired. Flexible and resilient, continuous learning systems evolve harmoniously with changes in the real world. This article explores the key principles of continuous learning, its applications, and the challenges and solutions facing the dynamic future of data.
What is Continual Learning?
Continual Learning (CL) is an approach in Machine Learning that can be translated as “continuous learning”. It is sometimes also called Incremental Learning or Lifelong Learning.
The idea behind CL is to mimic the human mind, where we continue to learn throughout our lives and where previously acquired information serves as the basis for new learning. Unlike traditional learning, where models are trained on a fixed set of data, continuous learning allows models to adapt and update continuously as new data becomes available, without having to start from scratch.
The aim of continuous learning is to enable models to be more flexible, adaptable and resilient in scenarios where data evolves or changes over time.
Let's talk about data sizes
It’s obvious that data is only going to continue to increase, that we have more and more of it every day, and that businesses need to be able to make use of it. So the question that naturally arises is how can we ensure that our Machine Learning models can process more and more data while remaining computationally efficient, or at least sustainable?
The answer is that we need to be able to process the data and then be able to get rid of it. Just as biological systems do: it would be impossible for a human being to process and save thousands of pieces of information over the long term!
So it’s important to think of Machine Learning models like the human mind, capable of filtering the information it picks up, retaining what’s important and building on that.
And even though computing power is only increasing, according to the IDC (International Data Group) we are on the point of exceeding the quantity of information generated by the capacity of the information we can store. By 2025, the rate of data generation will reach 160 ZO (zettabytes), of which we will only be able to store between 3% and 12%. This means that data will have to be processed on the fly, at the risk of losing it forever.
This is why continual learning is not only a way of optimising the computational load that models can represent by avoiding re-training them each time we have new data, but also a way of being able to process data before potentially losing it.
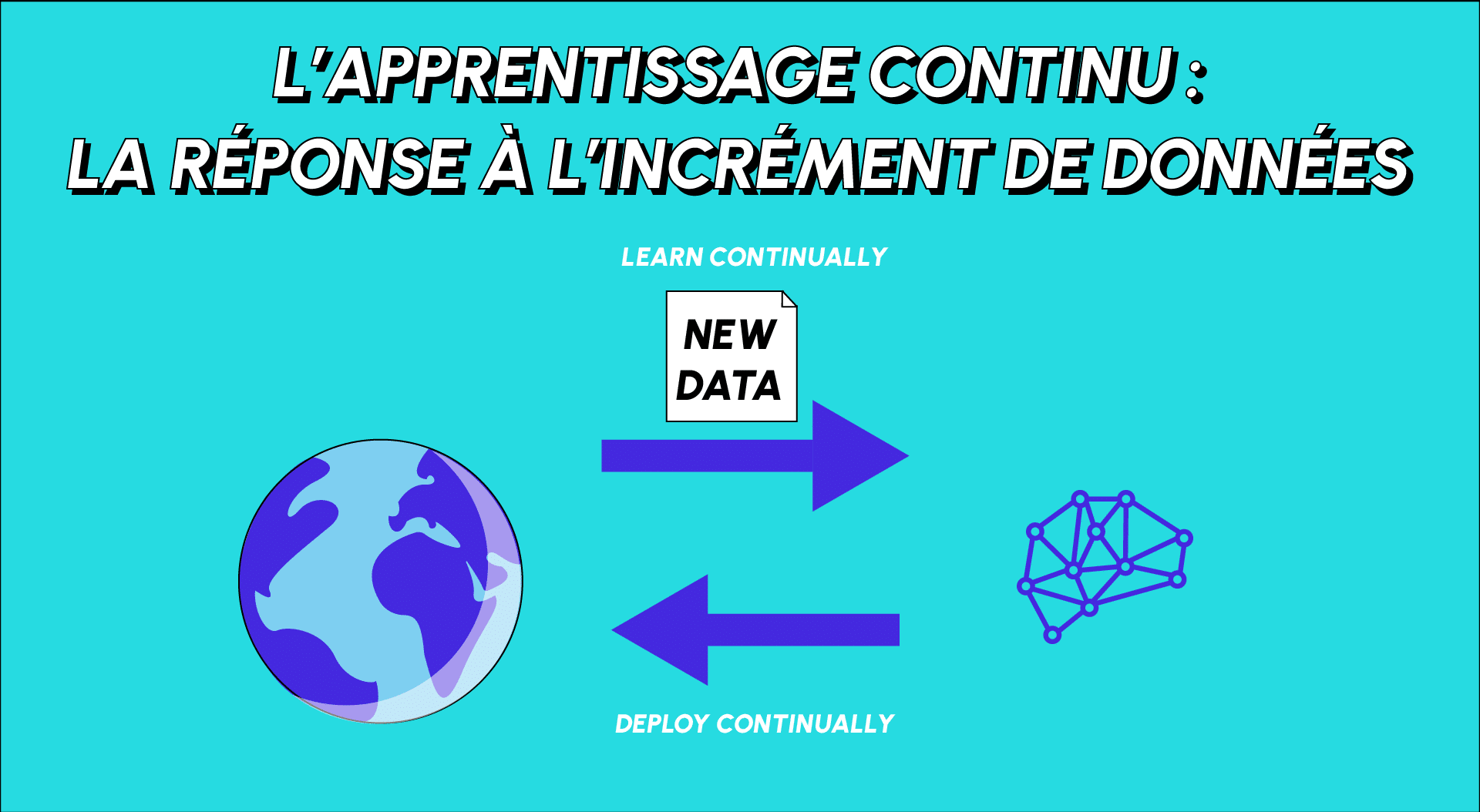
The main objectives
Having defined continual learning and discussed its benefits, its main objectives are as follows:
- Model flexibility. By enabling models to adapt continuously to new data, continuous learning makes models more flexible and better adapted to dynamic situations.
- Knowledge retention. Continuous learning aims to avoid catastrophic forgetting, a problem where models completely forget previously learned knowledge when updated with new data.
- Saving resources. Rather than training the model from scratch with the full set of data each time updates occur, continuous learning saves resources by making only incremental adjustments.
The challenges of lifelong learning
Even when continuous learning seems to be the ideal answer to dealing with the possible problems associated with data size in the future, as with everything in life, there are also a few challenges that need to be taken into account in order to obtain reliable, high-performance results.
- Catastrophic forgetfulness. When new data is presented, models must avoid forgetting previous knowledge. Approaches such as using external memories or re-training on old data with reduced weights for new tasks can help mitigate this problem.
- Fine tuning. To adapt the model to the new data, fine tuning of the weights is usually necessary. However, this can be difficult when there is heterogeneity of data and tasks. Adaptive fine-tuning or meta-learning techniques can be used to better manage this variability.
- Memory impracticality. In some cases, the amount of accumulated data can become too large to be stored in memory. The use of knowledge consolidation or intelligent sampling techniques can effectively manage this situation.
Some practical applications
Continuous learning has applications in various fields where data is constantly evolving. To mention just a few
- Speech recognition and natural language processing (NLP). NLP allows models to adapt to new expressions, words and concepts as they become commonplace.
- Computer vision. New images and videos are generated continuously. Continual learning allows models to update themselves to recognise new objects, scenes or other emerging visual features.
- Online learning systems. They can be updated based on user interactions, so continuous learning is essential to adapt machine learning models based on new information about user preferences and behaviours.
Conclusion
Continual learning is a very promising approach in Machine Learning for improving the adaptability of models to continuous changes in data and its growth. By allowing models to adjust gradually to new information, continuous learning opens the way to more flexible systems that are better adapted to dynamic environments.
Despite the associated challenges, recent advances in this field show that continuous learning is a promising direction for making Machine Learning systems more agile and efficient in a constantly changing world.