The root mean square error (RMSE) is an indicator for verifying the reliability of a model. This tool studies the discrepancies between the values actually observed and the values predicted by the model.
The squared error is a value that is always positive. The closer the values obtained with the model are to the observed values, the smaller the deviations and the closer to zero the squared error.
Square error is a positive value.
There are many other model validation indicators, such as coefficient of determination, bias and mean absolute error.
How is it calculated?
Its formula is as follows:
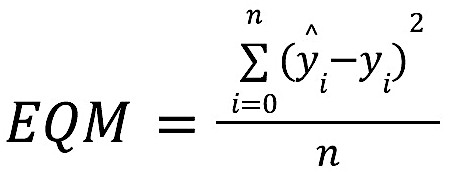
n: number of measurements
yi: values predicted by the model
yi : observed values
The root-mean-square error has several characteristics:
- It differs from other indicators in that it is easy to calculate.
- It is an indicator that is sensitive to outliers. In fact, by squaring the value of the deviations, each anomaly has a significant weighting in the calculation of the RMS error. To reduce the impact of anomalies, some use the root mean square as a model estimator.
- This can be difficult to interpret. Indeed, a root mean square error of 15 can be a guarantee of reliability if the mean of the observed values is greater than 1000.
- However, a root mean square error of 15 means that a model is highly unreliable if the mean of observed values is less than 100.
- So it’s always important to relate the root mean square error to the mean of observed values when judging a model’s reliability.
Application:
The blue dots represent observed values and the orange regression is the behavioral model established by the Data Scientist. Let’s calculate the mean square error of the model:
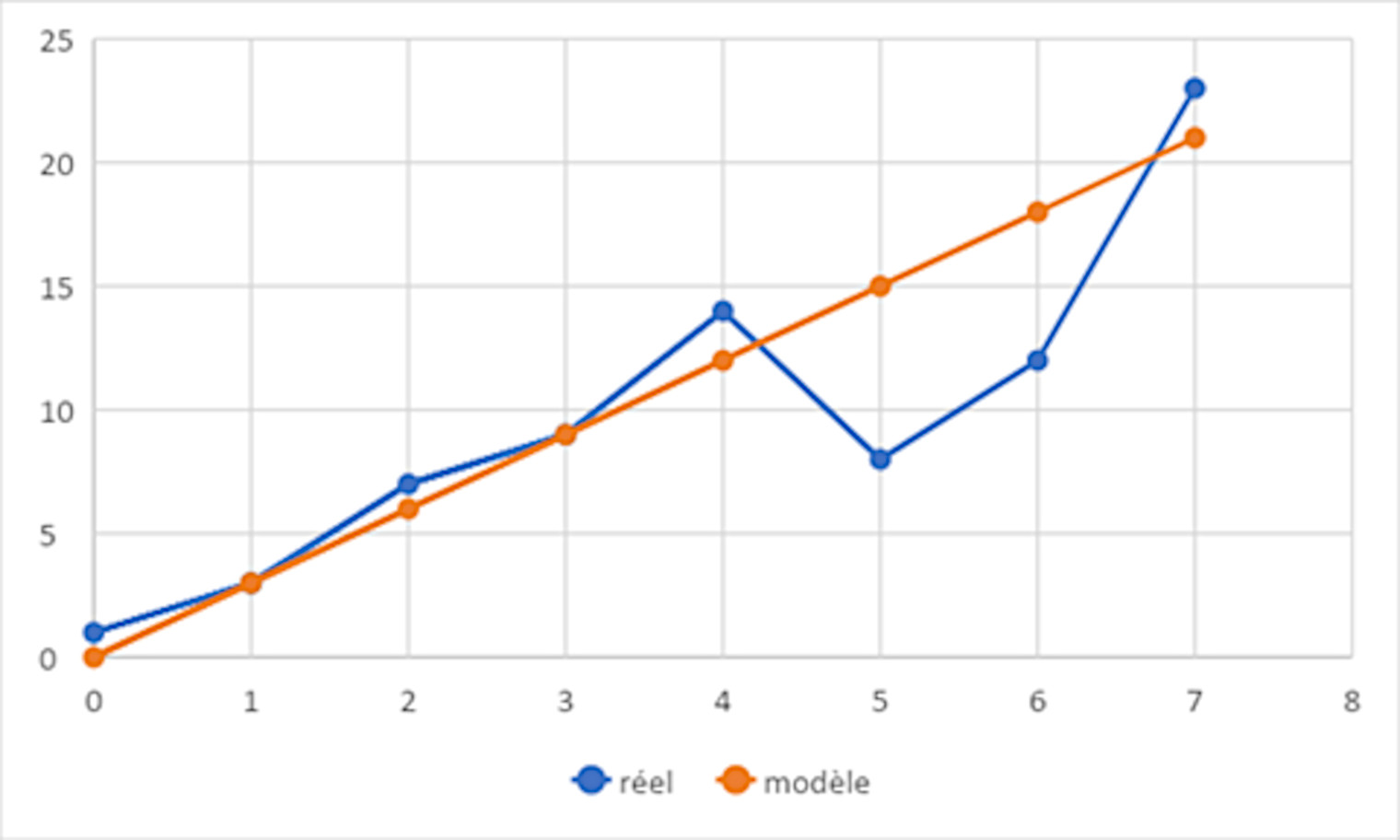
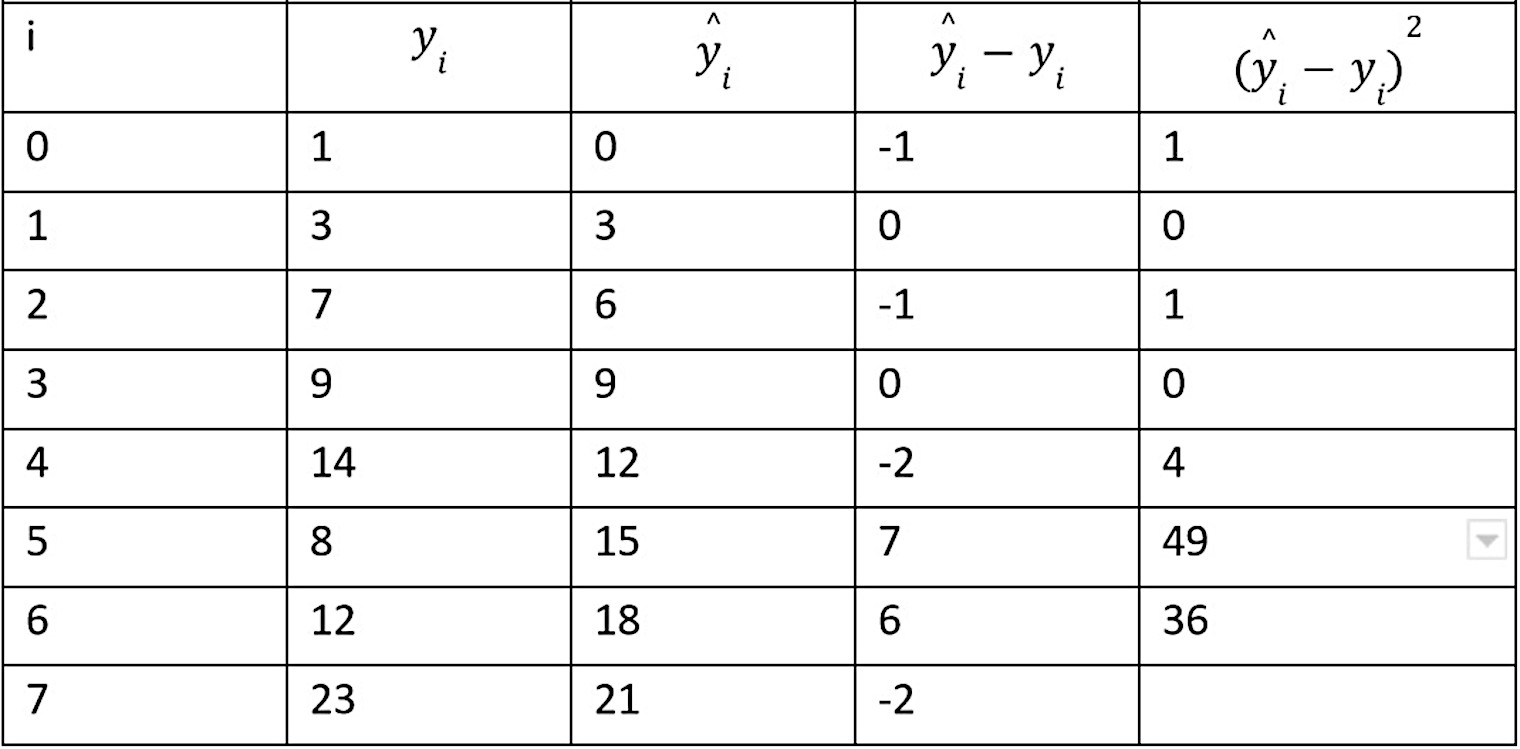

Where is it used?
The root mean square error, like other validation indicators, is being used more and more with the explosion in artificial intelligence and the growing development of predictive behavior models. Indeed, they are indispensable for verifying the relevance of a model.
It is therefore a tool used by data scientists, who are responsible not only for data analysis but also for implementing predictive algorithms within companies.
The root mean square error is already being applied in a wide range of fields, where the establishment of predictive models is essential. These include economics, with its stock market forecasting models, meteorology, with its atmospheric behavior models, and materials science, with its mechanical strength models.
How to control validation indicators?
As you can see, mastery of the various validation indicators is essential in the fields of Data Science and Machine Learning. To acquire these notions, and all the skills required for data science, you can opt for DataScientest.
Our professional training courses enable you to learn the Data Science professions such as Data Scientist, Data Analyst or Machine Learning Engineer. By the end of the course, you’ll have mastered programming, databases, Big Data frameworks, Machine Learning and DataViz.
Learners receive a diploma certified by the Université des Mines de Paris or the Université Paris Sorbonne, and are ready to enter the Data Science profession.
All our courses can be taken as Bootcamp or Continuing Education in a 100% distance learning format.
Don’t waste another moment and discover DataScientest’s training courses!