They say to err is human! But not only that. Intelligent machines can also make mistakes. This is known as AI hallucination. What are these hallucinations? What are the causes? What are the consequences? And above all, how can AI hallucinations be prevented? DataScientest answers your questions.
What is AI Hallucinations?
AI hallucinations are incorrect or misleading results generated by large language models. These LLMs are based on non-existent or poorly decoded training data, creating inaccurate or even “hallucinatory” results. This can be particularly problematic when it comes to making decisions.
Examples of AI hallucinations
Hallucination AIs affect all models, even the most sophisticated. Here are a few well-known examples:
- Google’s Bard chatbot claimed that the James Webb Space
- Telescope had captured the first images of a planet outside our solar system.
- Microsoft’s chatbot claimed to have fallen in love with its users and to have spied on Bing employees.
Beyond these examples involving the major players in artificial intelligence, LLM hallucinations often result in erroneous predictions, false positives or false negatives.
Positive applications
Even though AI hallucinations usually lead to an undesirable result, they may also hold some pleasant surprises in store for us. For example:
- The arts: these “errors” are particularly spectacular at the visual level. Learning models can produce “hallucinatory” images that allow us to develop a new approach to artistic creation.
- Data interpretation: even when producing erroneous results, the hallucinations of generative AI tools can reveal new connections and offer other perspectives.
- Games and virtual reality: AI hallucinations can help developers imagine new worlds.
- They can also add elements of surprise and unpredictability. All of which significantly enhances the experience for gamers.
That said, outside the creative field, AI hallucinations very often have harmful effects. But before looking at the dangers of these phenomena, let’s look at their causes.
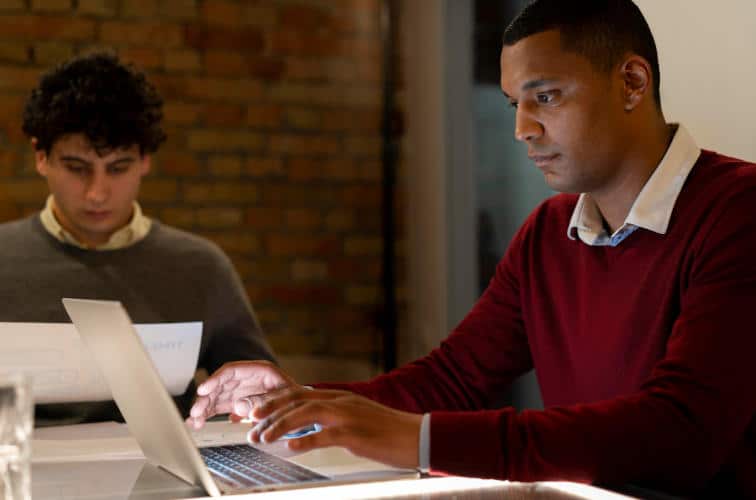
How can LLM hallucinations be explained?
In principle, LLMs train using data. This enables them to identify patterns in the data and make predictions. But a simple mistake in this training phase can have serious consequences for predictions.
Here are the most common errors that can cause AI hallucinations:
- Incomplete, inconsistent, out-of-date, inaccurate or biased training data;
- Poorly classified data;
- Biases in the data supplied to the models;
- Overfitting of data;
- Incorrect assumptions made by the AI model;
- Insufficient programming to prepare the model to interpret the information correctly;
- Lack of context;
- Model complexity.
Whatever the cause, the slightest error in preparing the models can completely distort future results.
What are the consequences of AI hallucinations?
With artificial intelligence now being used in a wide variety of sectors, AI hallucinations can have multiple harmful effects.
For example:
- In healthcare: an AI model could identify a serious illness, when it was actually a benign pathology. This leads to unnecessary medical intervention. The reverse is also true, resulting in no treatment for a patient who really needs it.
- In the field of security: AI hallucinations can disseminate erroneous information in highly sensitive sectors, such as national defence. Armed with inaccurate data, governments can make decisions with major diplomatic consequences.
- In finance: a model can identify fraudulent acts that are not really fraudulent, unnecessarily blocking financial transactions. Conversely, it can also miss certain frauds.
How can we limit the impact of AI hallucinations?
As the effects of AI hallucinations can be particularly harmful, it is essential to put in place all measures to limit them.
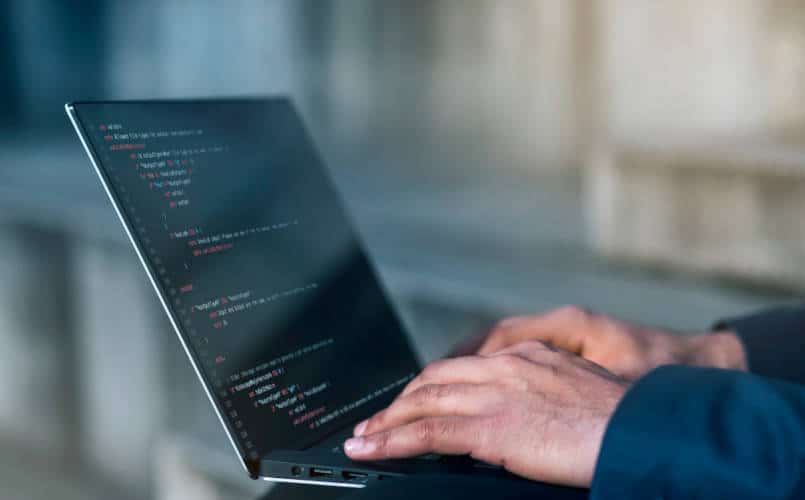
Taking care of your training data
The quality of the results from the large language model depends to a large extent on the quality of the training data.
Hence the importance of taking good care of it. To achieve this, it is necessary to carry out a meticulous preparation of the data. The idea is to ensure that no false, obsolete or inaccurate information pollutes the data set.
In addition to data quality, it is essential to provide the learning model with relevant data sets. In other words, data that is relevant to the task the model has to perform.
Finally, you also need to provide a large data set made up of diverse, balanced and well-structured data. This will minimise output bias, and therefore AI hallucinations.
Define your expectations precisely
To obtain a given result, you need to tell the artificial intelligence what you want, while defining the limits. In other words, specify what you want, and what you don’t want.
For example, with examples of well-written or badly-written texts if you want to create written content. You can also use filtering tools and/or probabilistic thresholds.
This way, the system will easily identify the tasks to be performed. The risk of AI hallucinations will then be reduced.
Using data models
Data models (or templates) are predefined formats designed to guide AI. By doing this, artificial intelligence is more likely to generate results that comply with your guidelines.
Once again, for a written text, your template can take up the basic structure:
- A title ;
- An introduction ;
- H2s (define the desired number);
- Paragraphs below each H2 (specify the number of words or a range);
- A conclusion.
As well as limiting AI hallucinations, the template also allows you to refine the responses of the artificial intelligence to produce a result that is more consistent with your expectations.
Continuously testing and refining the AI system
Even if you take precautions, AI hallucinations are commonplace. So to reduce their impact on decision-making, you need to rigorously test your AI model before using it. And above all, it’s vital to continue evaluating it, even after it’s been put into production.
As well as limiting the risk of error, this will improve the overall performance of the system and allow you to adjust the model as the data evolves.
Continuously testing and refining the AI system
Artificial intelligence can work wonders, but it must always be supervised by a human being. You will then need to examine each result provided. In the event of an error, this will enable you to identify the cause and correct it quickly.
Want to create AI models that are free from errors and hallucinations? Start by getting trained. At DataScientest, you’ll learn everything you need to know about data science, machine learning, deep learning… Join us!
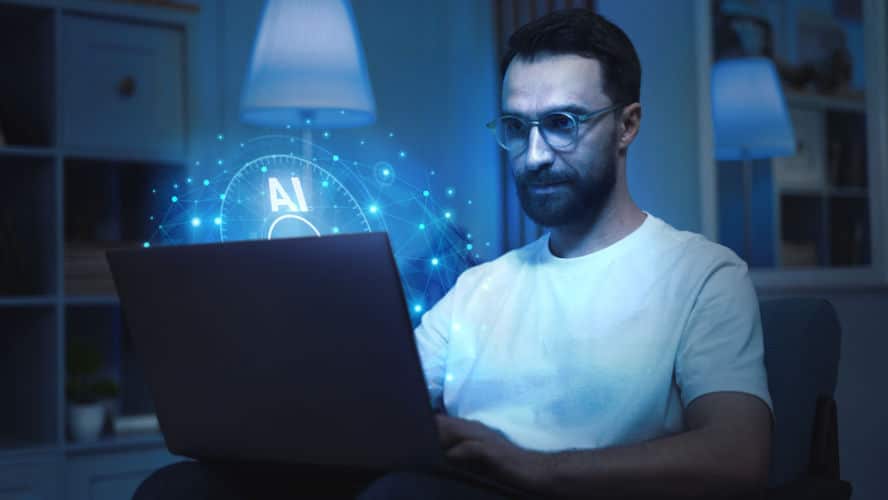