La divergence de Kullback-Leibler est une mesure de similarité entre deux distributions de probabilité, très utilisée pour l’analyse de données et le Machine Learning. Découvrez tout ce que vous devez savoir !
Our story begins in the United States in the 1950s. It was at this time that two American statisticians became interested in information theory and statistical analysis, and decided to look for a measure to quantify the difference between two probability distributions.
Born in 1907 in Poland, Solomon Kullback emigrated to the United States with his family during his childhood. He went on to study at the University of Michigan, where he obtained a doctorate in mathematics.
He subsequently worked as a statistician and researcher at various institutions, including the National Bureau of Standards and Columbia University.
Richard Leibler was born in New York in 1926. He obtained his doctorate in statistics at Columbia University, and has also worked in various research institutions. This specialist contributed in particular to the development of statistical methods for biology.
In 1951, in an article entitled “On Information and Sufficiency”, Kullback presented for the first time a concept that would forever mark the field of data analysis: the Kullback-Leibler divergence.
What is the Kullback-Leibler divergence?
It is a measure of similarity defined for two probability distributions, whether discrete or continuous.
Formally, the Kullback-Leibler divergence between two discrete probability distributions P and Q is given by “D(P||Q) = Σ P(i) * log(P(i) / Q(i))”.
However, the formula is slightly different for continuous probability distributions: “D(P||Q) = ∫ P(x) * log(P(x) / Q(x)) dx”.
This divergence is used to measure the dissimilarity between two distributions, in terms of missing information or the cost of representing one distribution using another. The greater the divergence, the more different the two distributions are.
What is the purpose of this analysis tool?
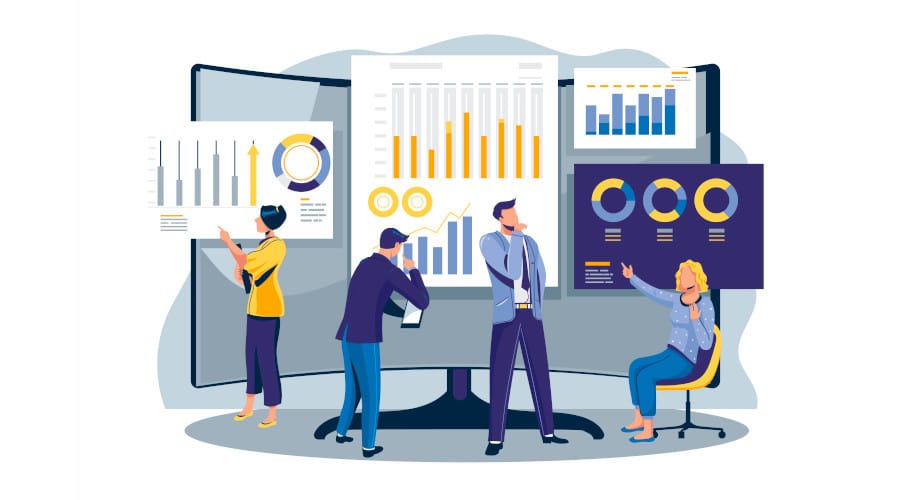
Almost a century after its invention, the Kullback-Leibler divergence is used in various areas of data analysis.
One of its most common uses is to measure model quality in classification and prediction problems.
By comparing the actual distribution of the data with the distribution predicted by a model, it can be used to assess how closely the model represents the observed data.
It can therefore be used to select the best model from several candidates, or to evaluate the performance of an existing model.
Another area of application is change detection. Kullback-Leibler divergence is used to compare probability distributions before and after a given event, to identify changes in time series.
For example, in environmental monitoring, it can be used to detect changes in pollution levels or variations in weather conditions.
It is also commonly used to compare empirical distributions. In sectors such as bioinformatics, it can be used, for example, to estimate the similarity between gene expression profiles.
This can be used to identify genes or biological pathways associated with specific diseases. Similarly, in information retrieval, it is possible to compare distributions of terms in documents to find relevant elements.
Conclusion: a comparison method for Data Analysts
Measuring similarity between probability distributions is an essential part of data analysis, and the Kullback-Leibler divergence is commonly used for this purpose.
By making it possible to evaluate the difference between two probability distributions, it quickly attracted the attention of researchers and is still a popular measure today in various fields such as data analysis and machine learning.
To learn how to master all the techniques and tools of data analysis and Machine Learning, you can choose DataScientest training courses.
Our various courses will give you all the skills you need to work as a Data Analyst, Data Scientist, Data Engineer, Data Product Manager or ML Engineer.
You will learn about the Python language and its libraries, databases, Business Intelligence, DataViz, and the various analysis and Machine Learning techniques.
All our training courses are distance learning, and you can receive professional certification thanks to our partnerships with prestigious universities and cloud providers such as AWS and Azure.
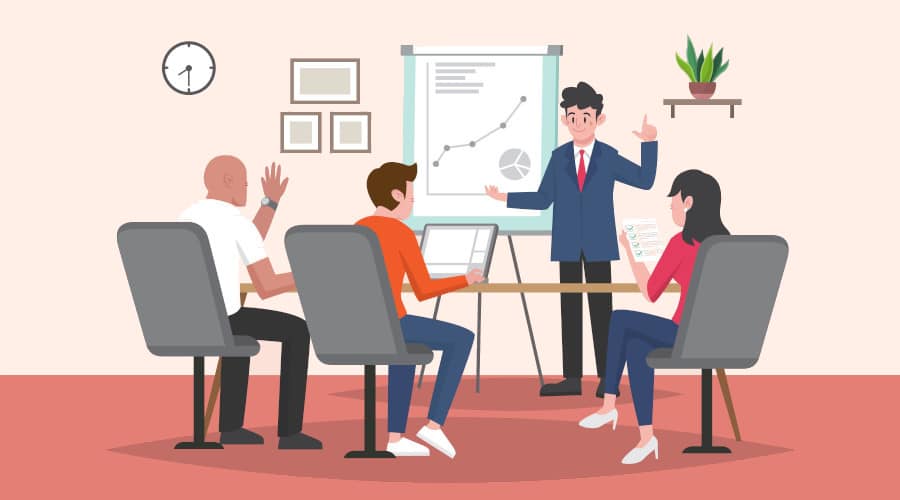